This Paper:Browse 244 Download 0 |
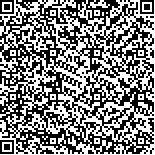 码上扫一扫! |
A hybrid data-driven and mechanism-basedmethod for vehicle trajectory prediction |
HaoqiHu1,XiangmingXiao2,BinLi1,ZeyangZhang2,LinZhang1,YanjunHuang1,HongChen3 |
|
(1 School of Automotive Studies, Tongji University, Shanghai 201804, China;2 Dongfeng Motor Corporation, Wuhan 430000, Hubei, China;3 College of Electronics and Information Engineering, Tongji University, Shanghai 201804, China) |
|
摘要: |
Ensuring the safe and efficient operation of self-driving vehicles relies heavily on accurately predicting their future trajectories.
Existing approaches commonly employ an encoder–decoder neural network structure to enhance information extraction during
the encoding phase. However, these methods often neglect the inclusion of road rule constraints during trajectory formulation
in the decoding phase. This paper proposes a novel method that combines neural networks and rule-based constraints in the
decoder stage to improve trajectory prediction accuracy while ensuring compliance with vehicle kinematics and road rules.
The approach separates vehicle trajectories into lateral and longitudinal routes and utilizes conditional variational autoencoder
(CVAE) to capture trajectory uncertainty. The evaluation results demonstrate a reduction of 32.4% and 27.6% in the average
displacement error (ADE) for predicting the top five and top ten trajectories, respectively, compared to the baseline method. |
关键词: Vehicle trajectory prediction · Rule knowledge · Graph attention network · Conditional variational autoencoder · Moving horizon optimization |
DOI:https://doi.org/10.1007/s11768-023-00170-x |
|
基金项目:This work was supported in part by the National Natural ScienceFoundation of China under Grant 52372393,62003238, and in part by the DongfengTechnology Center (Research and Application of Next-Generation Low-Carbonntelligent Architecture Technology). |
|
A hybrid data-driven and mechanism-basedmethod for vehicle trajectory prediction |
Haoqi Hu1,Xiangming Xiao2,Bin Li1,Zeyang Zhang2,Lin Zhang1,Yanjun Huang1,Hong Chen3 |
(1 School of Automotive Studies, Tongji University, Shanghai 201804, China;2 Dongfeng Motor Corporation, Wuhan 430000, Hubei, China;3 College of Electronics and Information Engineering, Tongji University, Shanghai 201804, China) |
Abstract: |
Ensuring the safe and efficient operation of self-driving vehicles relies heavily on accurately predicting their future trajectories.
Existing approaches commonly employ an encoder–decoder neural network structure to enhance information extraction during
the encoding phase. However, these methods often neglect the inclusion of road rule constraints during trajectory formulation
in the decoding phase. This paper proposes a novel method that combines neural networks and rule-based constraints in the
decoder stage to improve trajectory prediction accuracy while ensuring compliance with vehicle kinematics and road rules.
The approach separates vehicle trajectories into lateral and longitudinal routes and utilizes conditional variational autoencoder
(CVAE) to capture trajectory uncertainty. The evaluation results demonstrate a reduction of 32.4% and 27.6% in the average
displacement error (ADE) for predicting the top five and top ten trajectories, respectively, compared to the baseline method. |
Key words: Vehicle trajectory prediction · Rule knowledge · Graph attention network · Conditional variational autoencoder · Moving horizon optimization |