This Paper:Browse 236 Download 0 |
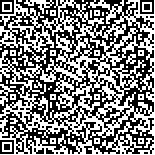 码上扫一扫! |
Heterogeneousmulti-player imitation learning |
BosenLian1,WenqianXue2,FrankL.Lewis3 |
|
(1 Department of Electrical and Computer Engineering, Auburn University, Auburn, AL 36849, USA;2 State Key Laboratory of Synthetical Automation for Process Industries and International Joint Research Laboratory of Integrated Automation, Northeastern University, Shenyang 110819, Liaoning, China;3 UTA Research Institute, University of Texas at Arlington (UTA), Fort Worth, TX 76118, USA) |
|
摘要: |
This paper studies imitation learning in nonlinear multi-player game systems with heterogeneous control input dynamics.
We propose a model-free data-driven inverse reinforcement learning (RL) algorithm for a leaner to find the cost functions of
a N-player Nash expert system given the expert’s states and control inputs. This allows us to address the imitation learning
problem without prior knowledge of the expert’s system dynamics. To achieve this, we provide a basic model-based algorithm
that is built upon RL and inverse optimal control. This serves as the foundation for our final model-free inverse RL algorithm
which is implemented via neural network-based value function approximators. Theoretical analysis and simulation examples
verify the methods. |
关键词: Imitation learning · Inverse reinforcement learning · Heterogeneous multi-player games · Data-driven model-free control |
DOI:https://doi.org/10.1007/s11768-023-00171-w |
|
基金项目: |
|
Heterogeneousmulti-player imitation learning |
Bosen Lian1,Wenqian Xue2,Frank L. Lewis3 |
(1 Department of Electrical and Computer Engineering, Auburn University, Auburn, AL 36849, USA;2 State Key Laboratory of Synthetical Automation for Process Industries and International Joint Research Laboratory of Integrated Automation, Northeastern University, Shenyang 110819, Liaoning, China;3 UTA Research Institute, University of Texas at Arlington (UTA), Fort Worth, TX 76118, USA) |
Abstract: |
This paper studies imitation learning in nonlinear multi-player game systems with heterogeneous control input dynamics.
We propose a model-free data-driven inverse reinforcement learning (RL) algorithm for a leaner to find the cost functions of
a N-player Nash expert system given the expert’s states and control inputs. This allows us to address the imitation learning
problem without prior knowledge of the expert’s system dynamics. To achieve this, we provide a basic model-based algorithm
that is built upon RL and inverse optimal control. This serves as the foundation for our final model-free inverse RL algorithm
which is implemented via neural network-based value function approximators. Theoretical analysis and simulation examples
verify the methods. |
Key words: Imitation learning · Inverse reinforcement learning · Heterogeneous multi-player games · Data-driven model-free control |