This Paper:Browse 302 Download 0 |
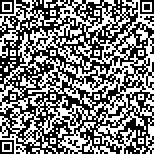 码上扫一扫! |
Dynamical pattern recognition for univariate time series and its application to an axial compressor |
JingtaoHu1,WeimingWu1,ZejianZhu2,CongWang1 |
|
(1 Center for Intelligent Medical Engineering, School of Control Science and Technology, Shandong University, Jinan 250061, Shandong, China;;2 School of Automation Science and Engineering, South China University of Technology, Guangzhou 510641, Guangdong, China.) |
|
摘要: |
In this paper, a learning and recognition approach is proposed for univariate time series composed of output measurements
of general nonlinear dynamical systems. Firstly, a class of dynamical systems in the canonical form is derived to describe the
univariate time series by introducing coordinate transformation. An observer-based deterministic learning technique is then
adopted to achieve dynamical modeling of the associated transformed systems of the training univariate time series, and the
modeling results in the form of radial basis function network (RBFN) models are stored in a pattern library. Subsequently,
multiple observer-based dynamical estimators containing the RBFN models in the pattern library are constructed for a test
univariate time series, and a recognition decision scheme is proposed by the derived recognition indicator. On this basis,
more concise recognition conditions are provided, which is beneficial for verifying the recognition results. Finally, simulation
studies on the Rossler system and aero-engine stall warning verify the effectiveness of the proposed approach. |
关键词: Dynamical pattern recognition · Deterministic learning · Stall warning · Radial basis function network · Sampled-data observer |
DOI:https://doi.org/10.1007/s11768-023-00193-4 |
|
基金项目:This work was supported by the National Postdoctoral Researcher Program of China (No. GZC20231451), the National Natural Science Foundation of China (Nos. 61890922, 62203263) and the Shandong Province Natural Science Foundation (Nos. ZR2020ZD40, ZR2022QF062). |
|
Dynamical pattern recognition for univariate time series and its application to an axial compressor |
Jingtao Hu1,Weiming Wu1,Zejian Zhu2,Cong Wang1 |
(1 Center for Intelligent Medical Engineering, School of Control Science and Technology, Shandong University, Jinan 250061, Shandong, China;;2 School of Automation Science and Engineering, South China University of Technology, Guangzhou 510641, Guangdong, China.) |
Abstract: |
In this paper, a learning and recognition approach is proposed for univariate time series composed of output measurements
of general nonlinear dynamical systems. Firstly, a class of dynamical systems in the canonical form is derived to describe the
univariate time series by introducing coordinate transformation. An observer-based deterministic learning technique is then
adopted to achieve dynamical modeling of the associated transformed systems of the training univariate time series, and the
modeling results in the form of radial basis function network (RBFN) models are stored in a pattern library. Subsequently,
multiple observer-based dynamical estimators containing the RBFN models in the pattern library are constructed for a test
univariate time series, and a recognition decision scheme is proposed by the derived recognition indicator. On this basis,
more concise recognition conditions are provided, which is beneficial for verifying the recognition results. Finally, simulation
studies on the Rossler system and aero-engine stall warning verify the effectiveness of the proposed approach. |
Key words: Dynamical pattern recognition · Deterministic learning · Stall warning · Radial basis function network · Sampled-data observer |